Extreme classification, where one needs to deal with multi-class and multi-label problems involving a very large number of categories, has opened up a new research frontier in machine learning. Many challenging applications, such as photo and video annotation and web page categorization, can benefit from being formulated as supervised learning tasks with millions, or even billions, of categories. Extreme classification can also give a fresh perspective on core learning problems such as ranking and recommendation by reformulating them as multi-class/label tasks where each item to be ranked or recommended is a separate category.
4th edition of the Large Scale Hierarchical Text Classification (LSHTC) Challenge.
The LSHTC Challenge was a hierarchical text classification competition, using very large datasets. We were happy to be involved in the winning team with Antti Puurula and Jesse Read.
http://arxiv.org/abs/1405.0546
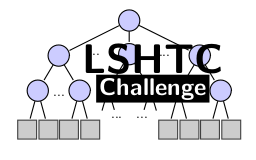
Hierarchies are becoming ever more popular for the organization of text documents, particularly on the Web. Web directories and Wikipedia are two examples of such hierarchies. Along with their widespread use comes the need for automated classification of new documents to the categories in the hierarchy. As the size of the hierarchy grows and the number of documents to be classified increases, a number of interesting machine learning problems arise. In particular, it is one of the rare situations where data sparsity remains an issue, despite the vastness of available data: as more documents become available, more classes are also added to the hierarchy, and there is a very high imbalance between the classes at different levels of the hierarchy. Additionally, the statistical dependence of the classes poses challenges and opportunities for new learning methods.
The challenge concerned multi-label classification based on the Wikipedia dataset. The hierarchy is a graph that can have cycles. The number of categories is roughly 325,000 and the number of documents is 2,400,000. A document can appear in multiple classes.
https://www.kaggle.com/c/lshtc